Choosing the right storage solution for AI
Explore your options for storage solutions for AI to learn which is right for your business.
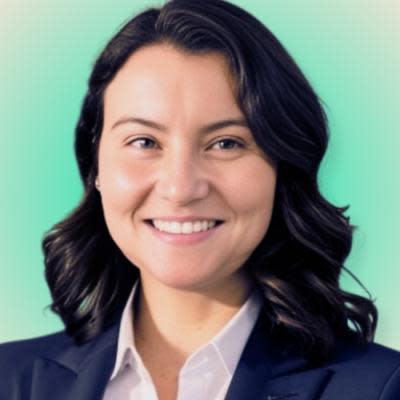
By Kelsie Anderson
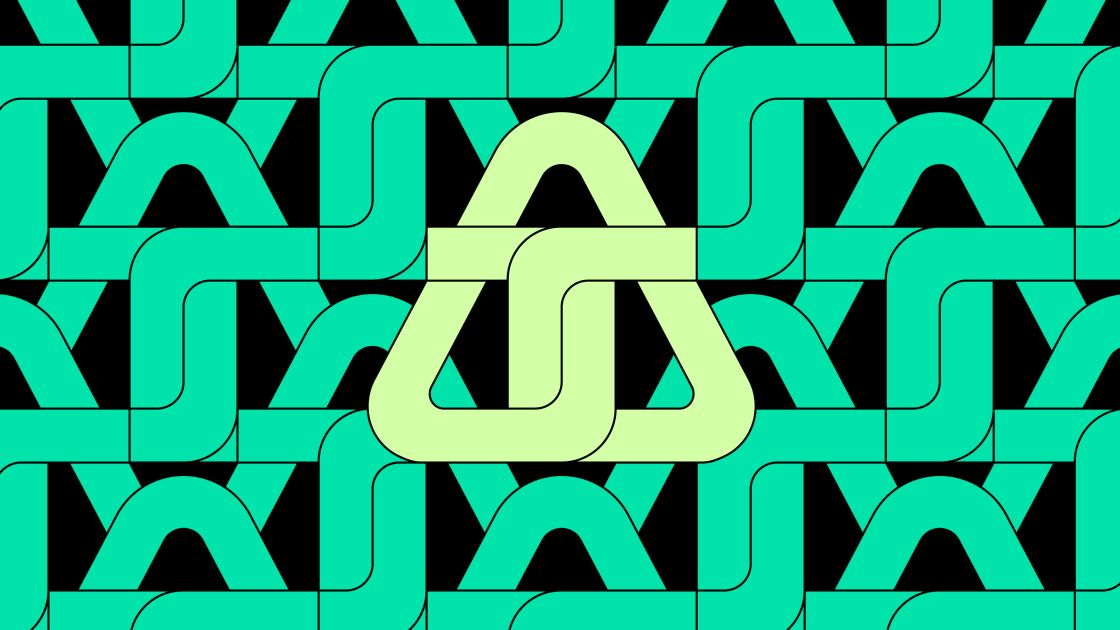
The recent surge of data-driven insights and groundbreaking algorithms in artificial intelligence (AI) is unprecedented. Today, nearly 80% of devices use AI in some way, and almost 85% of C-suite executives name AI as a key tool in driving growth.
With more and more applications leveraging AI, the quest for innovation is on. And at its core lies a pivotal component: storage. But your choice of storage for AI is about more than hoarding data. It’s about fueling the very essence of AI, enabling it to learn, adapt, and evolve.
The right storage solution is your gateway to harnessing the full potential of AI, bridging raw data and intelligent insights, unraveling mysteries within datasets, and propelling your projects to new heights. In addition to storing necessary data, storage built for AI has the speed, scalability, and adaptability to meet ever-evolving AI demands.
Your path to positioning your business at the forefront of AI innovation begins with a single step: choosing the right storage solution. Let’s take a look at the intricacies of AI storage solutions to unravel the characteristics that set them apart and guide you in making an informed decision tailored to your unique AI needs.
Understanding the storage demands of AI
AI applications, ranging from machine learning (ML) to deep learning (DL), need storage solutions that can handle vast volumes of data, deliver high throughput, offer low-latency access, and store data securely. Ultimately, the storage infrastructure must be able to support the iterative, data-intensive nature of AI workloads to facilitate seamless data ingestion, processing, and analysis.
High throughput and low latency
AI workloads require storage solutions that provide high throughput and low latency to accommodate the rapid processing of large datasets. High throughput ensures data can be read and written swiftly. Low latency guarantees quick data access, which is essential for real-time AI applications.
Scalability and flexibility
As AI models evolve and datasets grow, your storage infrastructure should scale accordingly. A scalable storage solution allows for the expansion of storage capacity and performance, ensuring the system can adapt to the increasing demands of AI applications.
Data durability and availability
Ensuring data durability and availability is crucial for AI applications. A robust storage solution must safeguard data against loss and provide continuous access to data—even in the event of hardware failures or other disruptions.
What needs to be stored for AI?
Several types of data and information need to be stored for AI applications. Each serves a distinct purpose in developing, training, and deploying AI models. Here are the key elements that require storage:
Raw data
Raw data is unprocessed, unfiltered information collected from various sources—analyst reports, Slack archives, product feedback—that serves as the foundation for training AI models. Depending on the application, this data can come in text, images, audio, video, or sensor readings.
Preprocessed data
Once raw data is collected, it often undergoes preprocessing to clean, normalize, and transform it into a suitable format for model training. Storing preprocessed data saves time and computational resources by avoiding redundant preprocessing steps.
Training datasets
Training datasets are subsets of preprocessed data used to train AI models. They’re labeled with the correct output, which the model learns to predict. Storing training datasets is crucial for refining models and evaluating their performance.
Validation and test datasets
Validation and test datasets are used to assess the performance of AI models during and after the training process. They help in tuning model parameters and avoiding overfitting, ensuring the model's generalization of unseen data.
Model parameters and weights
AI models consist of parameters and weights that are adjusted during training. Storing these elements is vital for preserving the trained state of the model, enabling further fine-tuning, and deploying the model for inference.
Model architecture
The structure or architecture of an AI model, including the arrangement of layers and nodes, needs to be stored for model reconstruction and deployment.
Hyperparameters
Hyperparameters are external configurations for the model training process, such as learning rate and batch size. Storing hyperparameters is necessary for replicating training conditions and experimenting with model optimization.
Feature engineering artifacts
Feature engineering involves creating new features from raw data to improve model performance. Artifacts from this process, such as feature selection criteria and transformation logic, need to be stored for consistency in model training and deployment.
Results and metrics
Performance metrics, evaluation results, and logs generated during training and testing are stored for analyzing model performance, diagnosing issues, and making improvements.
Inference data
Data used for making predictions, along with the resulting outputs, may be stored for auditing, monitoring model performance in production, and further refining the model.
Embeddings
Embedding refers to a technique used to represent discrete categorical data—such as words, sentences, or identifiers—in continuous vector spaces. They’re essential for converting symbolic data into a form that can be processed by machine learning models, particularly neural networks.
Embeddings are widely used in natural language processing (NLP), recommendation systems, and various other AI applications. Storing data as vectors can reduce the time taken for inference.
Code and scripts
The codebase, scripts, and notebooks used for data preprocessing, model training, evaluation, and deployment are stored for version control, collaboration, and reproducibility.
Documentation and metadata
Documentation detailing the development process, model architecture, and usage—along with metadata describing the datasets and model configurations—is stored for reference, compliance, and knowledge sharing.
By systematically storing these elements, organizations can ensure the robustness, reproducibility, and continuous improvement of AI applications while adhering to best practices for data management and compliance.
Evaluating storage options for AI
Clearly, AI requires storing tons of data. So, a key piece of fast generative AI is quick access to large datasets. But deep learning algorithms such as large language models (LLMs) are too, well, large to run on memory alone. That’s where stored data becomes necessary.
Several storage options cater to the diverse needs of AI. Evaluating these options involves considering factors such as performance, scalability, cost, and the specific requirements of the AI workload. We’ll take a look at some common choices below.
Object storage
Object storage is a scalable, cost-effective solution ideal for storing large volumes of unstructured data. It offers high durability and availability, making it suitable for AI applications that require long-term data retention and access.
Block storage
Block storage provides low-latency, high-performance access to data, making it well-suited for AI workloads that demand rapid data processing. It’s typically used for structured data and is ideal for applications like databases and file systems.
File storage
File storage offers a hierarchical structure for organizing and accessing data. It’s versatile and can support both structured and unstructured data, making it a viable option for various AI applications.
Cloud storage
Cloud storage solutions offer scalability, flexibility, and a pay-as-you-go pricing model. They provide a range of storage options, including object, block, and file storage, allowing organizations to choose the best fit for their AI workloads.
Best practices for implementing AI storage solutions
Implementing the right storage solution for AI involves adhering to best practices that optimize performance, scalability, and cost.
Assess workload requirements
Understanding the specific requirements of your AI workload is fundamental. Assess the volume of data, the need for high throughput and low latency, and the scalability and availability requirements to select the most suitable storage solution for your application’s needs.
Leverage hybrid storage solutions
As AI becomes key to business performance, a hybrid approach—which combines on-premise and cloud storage—can offer the best of both worlds. It allows organizations to experience the high performance of on-premise storage while benefiting from the scalability and flexibility of cloud storage. It also provides failovers to aid in disaster recovery.
Ensure data security and compliance
Safeguarding data is paramount. Implement robust security measures, including encryption and access controls, and ensure compliance with data protection regulations to protect sensitive information.
Monitor and optimize
Regularly monitor the performance of your storage infrastructure and optimize it to meet the changing demands of your AI applications. Proactive monitoring and optimization can prevent bottlenecks and ensure efficient functioning of your AI workloads.
Level up your AI strategy with the right storage solution
Choosing the right storage solution for AI is a nuanced decision that involves evaluating various options and aligning them with the specific needs of your AI workloads. By understanding the storage demands of AI, assessing different storage options, and adhering to best practices, you can implement a storage infrastructure that optimally supports the growth and evolution of AI in your applications.
Navigating the multifaceted landscape of AI storage solutions can be overwhelming, but the rewards are worth the effort. By finding a solution that works for your AI application, you can position your business at the forefront of AI innovation by meticulously evaluating your AI application’s unique requirements and aligning them with a storage solution that offers the optimal blend of speed, scalability, and adaptability.
But staying ahead of the curve involves more than embracing AI. You have to bolster your AI strategy with the right tools and infrastructure. The choice of storage solution you make today will shape the trajectory of your AI projects, influence the insights you glean, and determine the impact you make. It’s a decision that calls for foresight, discernment, and a deep understanding of the symbiotic relationship between AI and storage.
Telnyx’s Storage combined with our Inference tool helps you achieve that symbiosis in one platform, giving you end-to-end control over your AI products. Our low-cost, low-latency storage solution gives you quick access to affordable data storage. Equipped with our AI embed, you can vectorize that data in seconds to build and train fast iterative AI applications. With Inference, you can leverage that custom data in proprietary and open-source models or build your own on dedicated GPU infrastructure for fast inference at low costs.
Our competitive pricing for Storage and Inference makes our tools accessible to small teams and enterprise businesses. With pay-as-you-go structures, you only pay for what you need, allowing you to scale as your AI needs grow. And our award-winning customer service team is available 24/7 for any questions you might have.
Contact our team to learn how you can use Telnyx Storage and Inference to create next-gen AI applications for your business. Or try the solution for yourself by signing up for a free Telnyx account.
Sign up for emails of our latest articles and news
Related articles