Conversational AI vs. generative AI: Key differences
Understand the differences between conversational and generative AI and how to leverage them for your business.
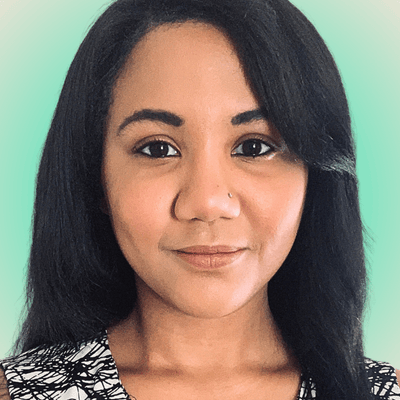
By Tiffany McDowell
The standard tech stack for businesses has a new normal. Artificial intelligence (AI) has become an increasingly significant area of interest for companies seeking a competitive advantage. Within this field, two terms frequently garner attention and curiosity: conversational AI and generative AI.
These technologies are crucial components of the tech landscape, each with its own set of capabilities and applications. Both offer a boost in productivity and a reduction in costs when used correctly. By understanding the key features and differences of each, you can maximize the benefits to your bottom line.
Learn the nuances between conversational AI vs. generative AI to better understand how and when to use them in your organization.
What is generative AI?
Generative AI encompasses a wide range of AI technologies. Its focus is on creating new content—whether it be text, images, music, or any other form of media. Unlike conversational AI, which is designed to understand and respond to inputs in a conversational manner, generative AI can create entirely new outputs based on the training data it’s been fed. For example, generative AI can create new marketing content by learning from past successes and replicating effective patterns. This ability is particularly valuable in dynamic fields like marketing, design, and entertainment.
Generative AI is incredibly powerful for a variety of applications, and can be defined by several key features:
Creativity and deep learning
Generative AI harnesses its ability to think outside the box, generating content that can surprise and inspire, often mimicking human creativity. It’s continuously evolving and improving its output by learning from extensive datasets to mimic human-like creation.
Personalization
Any content generated is based on specific parameters or preferences. This feature allows generative AI to customize its output to meet the unique needs and preferences of individual users, enhancing user engagement and satisfaction.
Machine learning models
These are at the heart of generative AI, with models like GANs (Generative Adversarial Networks) and transformers being particularly prominent. These models serve as the backbone of generative AI, driving its ability to generate realistic and diverse content across various domains.
Applications of generative AI
Generative AI has great potential in many areas. It can create original content in fields like art and literature, assist in scientific research, and improve decision-making in finance and healthcare. Its adaptability and innovation promise to bring significant advancements across various domains.
Common examples of popular generative AI applications include ChatGPT, Google Bard, and Jasper AI. Additional applications include:
- Content creation: Generates articles, stories, and scripts for media outlets, marketers, and content creators.
- Design: Creates artwork, logos, and graphics for branding and marketing purposes.
- Language translation: Transforms text between languages with improved accuracy and fluency.
- Better chatbots: Enhances customer interactions with natural, intelligent, and responsive conversation capabilities.
What is conversational AI?
Conversational AI refers to AI systems designed to interact with humans through natural language. The core purpose of conversational AI is to facilitate effective and efficient interaction between humans and machines using natural language.
It’s important to note here that conversational AI often relies on generative AI to conduct these human-like interactions. For example, when you pose a question to a conversational AI system, it passes that input to a large language model (LLM) to form an output or response. In this case, the output is generated by an LLM based on trained data.
Conversational AI powers chatbots, virtual assistants, and voice-activated devices and can be defined by several key features:
Natural language processing (NLP)
NLP combines rule-based modeling of human language with statistical, machine learning, and deep learning models. This process allows conversational AI systems to understand and interpret human language, resulting in more natural and meaningful interactions between humans and machines.
Multi-channel support
Conversational AI enables interactions across various communication channels, including messaging apps, websites, and voice interfaces. This feature ensures that users can engage with conversational AI systems through their preferred channels, enhancing accessibility and user experience.
Speech recognition and synthesis
This feature allows conversational AI to interact verbally by recognizing human speech and responding in kind. Speech recognition converts spoken language into text that the AI system can understand, while speech synthesis generates human-like speech from text responses, enabling seamless verbal communication between humans and machines.
Applications of conversational AI
Conversational AI has a variety of applications spanning a multitude of industries. Common applications of conversational AI encompass widely recognized platforms such as Alexa, Google Assistant, and Siri. As a result, many companies have optimized their customer service experience and sales pipelines. Some industries that have benefited include:
- Customer support: Chatbots and voice assistants equipped with conversational AI streamline customer inquiries, providing instant assistance and resolving issues efficiently.
- E-commerce: Virtual shopping assistants enhance the online shopping experience by guiding customers, recommending products, and processing transactions.
- Healthcare: Conversational AI aids in patient engagement, appointment scheduling, and medical diagnosis through virtual healthcare assistants.
Ultimately, the adoption of conversational AI technology has elevated customer satisfaction and propelled businesses toward greater efficiency and competitiveness in the current market landscape.
Conversational vs. generative AI: What’s the difference?
While both conversational AI and generative AI contribute to enhancing user experiences and driving innovation, they differ in a number of ways:
Conversational AI | Generative AI | |
---|---|---|
Purpose | Facilitating human-like conversations | Generating content across various formats |
Use cases | Customer service, virtual assistants, AI chatbots | Creating fiction, marketing materials, meta descriptions |
Datasets | Trained on extensive datasets comprising human interactions, conversations, user queries, and responses | Learns from diverse datasets to identify patterns for generating content with predictive elements |
Input and output | Users input information during conversations, which the system then uses to formulate responses | Utilizes input and learned patterns to generate fresh content |
Again, it’s important to note that many conversational AI tools rely on generative AI to create their human-like responses. So while there are differences between the two technologies and the processes they use, they’re not mutually exclusive.
Embracing the AI variations
In the thriving field of AI, both conversational and generative AI have carved out distinct roles. Conversational AI tools used in customer-facing applications are being developed to have more context on users, improving customer experiences and enabling even smoother interactions. Meanwhile, more general generative AI models, like Llama-3, are poised to keep pushing the boundaries of creativity, making waves in artistic expression, content creation, and innovation.
Understanding these distinctions is key to leveraging their power effectively. Whether enhancing customer interaction or sparking innovation, both AI types offer transformative advantages for industries.
Leverage conversational and generative AI with Telnyx
Adopting AI is essential for meeting customer expectations and staying competitive. AI has the potential to change the way we work on a global scale. But for that to work, it needs to be reliable, flexible, and scalable to accommodate business needs. Telnyx recognizes the intricacies involved with AI adoption and is equipped to navigate these complexities.
We built our LLM library to give our users options when choosing which models to build into their applications. For example, you can use Llama 3 for text, image, and video processing and Google Gemma for great text summarization and Q&A. Telnyx Inference can use data from Telnyx Cloud Storage buckets to produce accurate, contextualized responses from LLMs in conversational AI use cases.
From revolutionizing customer engagements through conversational AI bots to advancing other generative AI processes, Telnyx is committed to delivering tangible, dependable results. We're on a mission to make AI more than just another complicated tech stack. We want to provide a genuinely accessible, valuable tool to businesses of any size. Leveraging our global infrastructure and a suite of user-friendly tools tailored for real-world applications, you're empowered to harness AI's full potential for your applications.
Contact our team to learn how our advanced solutions can help you stay ahead in an increasingly AI-automated world.
Sign up for emails of our latest articles and news
Related articles